The false call killer
— a Q & A with Romain Roux and Nicolas Guillot on DeepReview and the power of AI-driven inspectionSome call it the “false call killer”: a new Automatic Defect Classification system that leverages deep learning to dramatically reduce false call rates while improving first-pass yield in 3D Automated Optical Inspection (AOI). The recent release of DeepReview comes as welcome news for manufacturers applying full test coverage, where any variation in material, component type or board design can unleash new streams of tedious false calls. To learn more about DeepReview and the benefits of deep learning in the daily lives of review operators, we spoke to two of the system’s key developers: Romain Roux, R&D Director for AI, and Nicolas Guillot, Software Systems Architect.
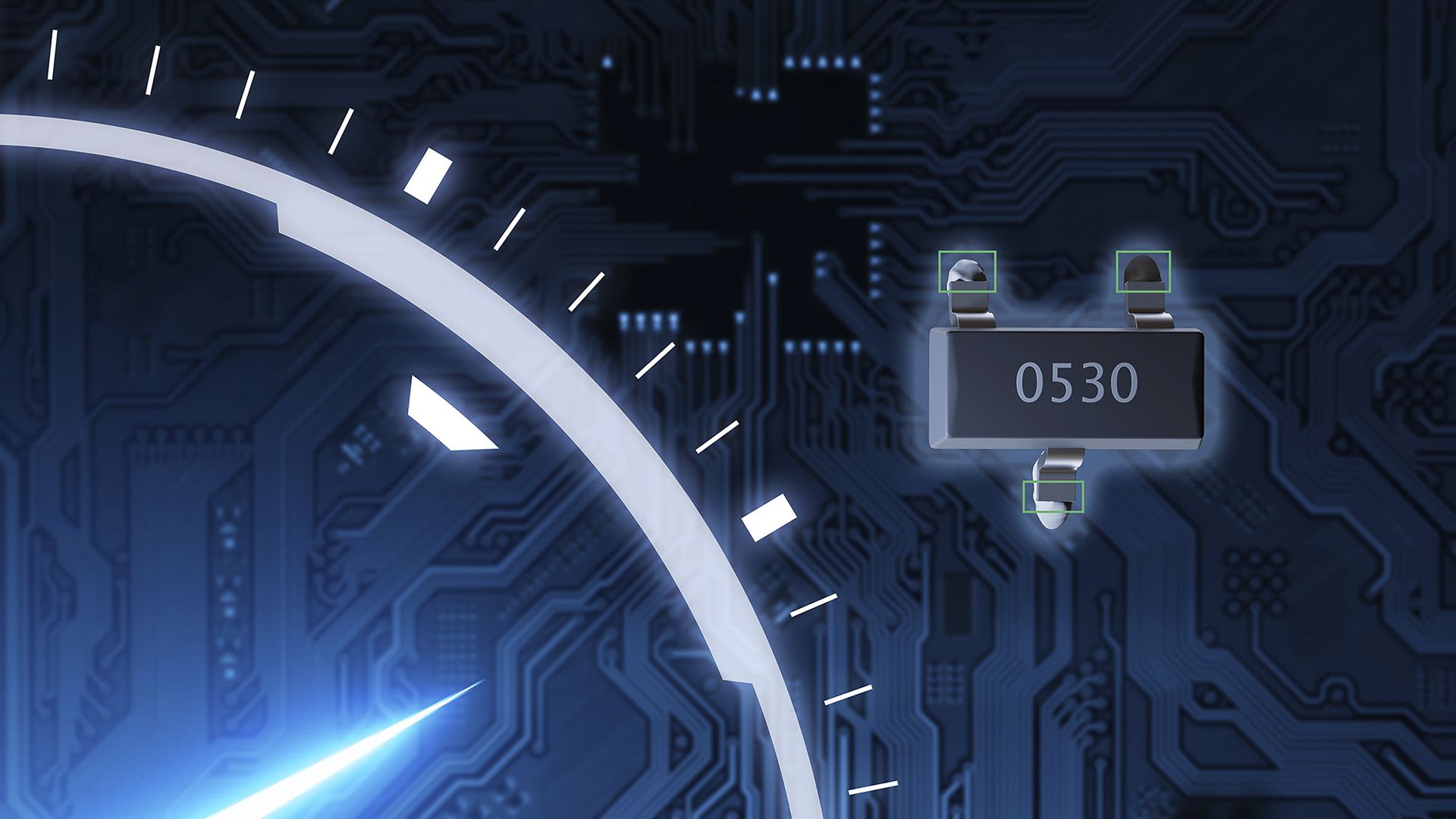
Where did the idea for DeepReview come from?
Romain: The concept came from a collaboration with a customer who shared some AOI images and corresponding certifications with us. At the time, I was at the Center for Deep Learning in Electronics Manufacturing (CDLe), a five-year joint industry initiative in San Jose, California. I was surrounded by some of the best experts in the field, and we wanted to see what kind of customer value could be generated by applying deep learning to the massive amounts of high-quality inspection data that all our customers are sitting on. Since image classification is one of the most mature applications within deep learning, it didn’t take long to build a good neural network.
So, was this a model that could be applied directly in production?
Romain: Maybe for this customer, but it wouldn’t have worked for anyone else. There are so many variables like the design, color and type of component, which all complicate classification. Even between customers, tolerances differ. Some would want to repair a particular issue. Others wouldn’t. And this is the core challenge: A generic model is extremely difficult to create. Every customer has their own standards, their own supply chain and process variations, and only they are completely familiar with what quality standards are acceptable.
Romain Roux R&D Director for AI, (left).
Nicolas Guillot Software Systems Architect, (right).
Have you met customers who’ve tried to develop their own AI classification systems? If every neural network needs to be customized, why don’t they just build their own from scratch?
Romain: Sure, there are standard algorithms out there. But the ramp-up is daunting. You have to develop the tool, label the data, learn how to use the library, connect to the AOI system, etc. It could easily be a half-million-dollar project, and even then you’d have to be a data scientist to use it. Instead, we’ve built the brain and an interface that speaks inspection language, rather than specialized image processing language. So customers can just do a little labeling, which they already do when reviewing AOI defects, and focus on what they’re best at: stopping real defects.
Nicolas: This is really the key point. Our customers already have everything they need to use DeepReview. They’ve got years of actual production data. They’re already labeling, classifying and performing sanctions on false calls and true defects. We’ve focused our efforts on building a deep learning system that learns from what they’re already doing, so a person doesn’t keep having to do the repetitive jobs that a machine can do. They shouldn’t have to babysit a machine and constantly sanction false calls, and they definitely shouldn’t need a PhD in image processing or AI. They should be out there kitting, dekitting and doing repair, where their expertise is needed most!
50-90% fewer false calls is a realistic expectation.
So, what kind of time savings are we talking about? How many false calls can customers expect to cut out of their daily work?
Nicolas: It really depends on the variety and types of components, but 50-90% fewer false calls is a realistic expectation. With DeepReview, an operator can definitely feel confident that they’re more pro-ductive, with less risk for errors. But there’s more to it than that, since the system continually improves the inspection process. If before you had, say, 10 AOI defects including 8 false calls and 2 real defects, the system might automatically reduce that to just 2 defects for review. So, as it works in the background, it’s improving the robustness of the inspection model itself.
It’s great that a customer doesn’t need to learn data science to understand how deep learning does its job, but how do they know they can trust it to make the right calls?
Nicolas: This is a super important point, and there are mainly two answers to that. The first is that the system is trained on the customer’s own historical production data, their own images and their own sanctions. So, it’s only the manufacturer’s own tolerances that enter into the system, meaning there’s no risk of introducing new escapes. The second is that the annotation and neural network training steps are designed to give users an understanding of the impact of their inspection model — it’s like peeking inside the box. The interface is super simple to use, it lets you easily group similar images to find any problems and validate all the others in one click. A half-hour of training is enough, and then everything else — the data storage, importing, converting, etc. — are all automated.
AOI inspection process with DeepReview.
And what about data security? This is pretty sensitive information to put into the hands of an AI…
Romain: Since the server is on-premises, running in a closed system on the customer’s own sub-network, all data collection, storage, training and processing are done locally. The customer controls everything. It’s their own neural network, their own AI server.
Finally, for those who’ve heard a lot of promises about AI recently, exactly what kinds of features can DeepReview recognize? And what are its limitations?
Romain: I totally agree that it’s important to separate the reality from all the hype that’s out there around AI. It’s crucial to stay realistic. With DeepReview today, everything that can be seen in 2D color imaging on smaller components — damaged, rotated, tilted or misaligned components, or bad solder joints, for example — can all be processed. And additional capabilities — like the ability to analyze each lead of a QFP or bigger component — are coming soon.
Nicolas: The limitations have more to do with the limits of image classification in general — the fact that even the most advanced AI models in the world struggle with classifying complex patterns in 3D — rather than the limits of our particular system. But AI is evolving so fast that even this will change. And that’s one of the advantages of the annotation process within DeepReview: Everything a customer does is effectively tagging the images in a way that’s designed for the AOI to understand. So there are a lot of potential benefits even for the pick-and-place, solder paste Jet Printer and SPI in the future. This means that when new tasks arise, our customers will be ready. And everything will be so much easier with a complete library, fully annotated and sanctioned by the people who know best: the PCB manufacturers themselves.
DeepReview AI-guided labelling and training interface.